Artificial Intelligence: Why Regulators Need to Understand Startups First
We all love to reflect on the big questions in life while driving. This makes us great thinkers and bad drivers.
Entrepreneurs and scientists are the rockstars of this century. Artificial Intelligence is exploding around the world and in Europe. In this wave of WTF? and OMG we want to look at how Europe can keep up while others declare world leadership. Other than popular belief we want to argue that the main drivers of AI and machine learning technologies are startups, not corporates. And hence that governments and regulators need to think startup-first. Startups rethink and oftentimes bend our imagination of what we thought was normal or even possible — unless prohibited by regulation. Hear our pledge to understanding AI through startups.
What Governments should know about AI startups, and what startup founders should be asking for when speaking to regulators?
Europe is in the driver’s seat of deep tech and hosts a whopping array of AI startups. Investments in AI have become number 1 in terms of volume and number of deals, which makes artificial intelligence the main vertical for EU startups. Atomico’s State of European Tech makes a pounding case. Still, not everyone is aware that Europe has the potential to take a large piece of the global AI-cake of over 15 trillion $. We have the talent, the companies and the data to succeed. But how will Europe’s regulatory framework help us get there rather than drive companies away?
The political debate around AI still seems off focus and, as the Commissioner for Innovation recently stated, dominated by critics, alarmists and hysterics.AI deserves a shift in perspective, or perhaps even a paradigm shift. First, rather than falling for the anthropomorphic science-fiction logic of killing robots, it should be clarified what AI is. We should figure out where we currently lie on a scale between arithmetic and singularity. Second, AI cannot be discussed without a ‘think startups first’ approach. As we will show, startups is where most AI starts. Thirdly, just as Commissioner Moedas, we want to move away from the hysteria and tell the real story.
A nimble and flexible policy approach to AI
The perception and definition of AI has always been fluid. John McCarthy said in the 1950s that “as soon as it works, no one calls it AI anymore”. In the early 2000s, systems that analysed and found patterns in data were called text and data mining (TDM).
The closest that we come to an classification is probably looking at narrow versus general versus super AI. Narrow AI is where we stand today — systems that learn how to perform one specific task, and only that. That is the case when machines play go, recognise language or make sales predictions. Worrying today about artificial super intelligence is, in the words of Andrew Ng, like “combating overpopulation on planet Mars”.
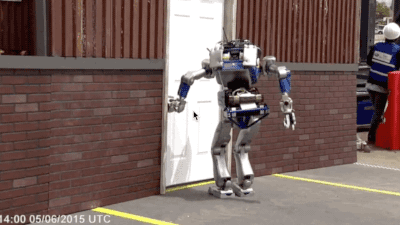
Rather than looking into what will or might be possible in the future, policymakers should look at AI today. Today’s most popular AI-fueled applications aren’t ‘intelligent’ as such, but rather augment and assist human intelligence to make decisions and predictions. In recent history, machine automation in 80s and 90s showed us that dishwashers, washing machines and land movers can perform some of the tasks that humans do, but in a different way rather than exactly replicating the human processes.
Today’s examples revolve around navigating traffic, spell checking, suggestions and data analytics. Say again, Siri? AI is not fairy dust sprinkled on a stack of data to turn it into gold. It’s one tool in the hands of developers creating a service or a product. This may be less exciting than talking about replicants à la Blade Runner, but facts and evidence make a better basis for law. For everything we don’t know, we should retain a healthy portion of flexibility in our legislative framework. For everything we do know, we should ask whether it’s broken before trying to fix it.
Can there be artificial intelligence without startups?
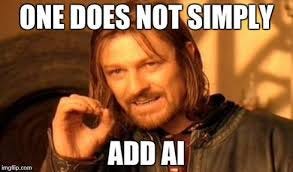
One of the popular and often cited trends in today’s digital economy is that large companies keep tabs on the market with all the data they hold. The fact is that most of the technology is acquired through startups. The number of mergers and acquisitions around AI has been rising steadily from only two in Q1 2012 to staggering 37 in Q1 2017. It is worth clarifying that exits — startups being acquired by larger companies — are good for the entire ecosystem, not only for the entrepreneurs who make the deal. They are quite unlikely to spend the rest of their life on an island sipping cocktails. Far more likely, entrepreneurs will reinvest capital and expertise in other startups. The same holds true for co-founders and early employees. Together with fresh money for the investors, they keep the funding wheel turning. And in the end, the acquiring company will have a better product, which benefits customers.
Some of the most popular applications, such as image recognition, natural language processing, recommendations or automated driving, haven’t been invented or driven forward in corporations but in Startups. Small teams either brought their technology and know-how into another company or grew it on their own. Most recent examples include Amsterdam-based ReSnap, Scyfer or Sonalytic. Or Niland, where former startup Spotify illustrates this lifecycle. Most popular examples are listed here and include Google’s DeepMind, Uber’s OTTO or Amazon’s angel.ai.
Those AI startups generally do not operate in the more visible consumer facing space as stand-alone applications. But there are many startups that use AI tools to provide their main service or product. As futurist and author Kevin Kelly said: “The business plans of the next 10,000 startups are easy to forecast: take X and add AI” .
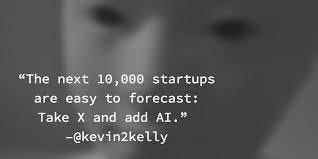
Overall, we observe a steep rise in company creation. Investment in the sector shows the overall health and growth potential of the AI market. Gartner’s Hype cycle suggests that peaks for machine learning and deep learning will be only be reached after 2020. Europe is on track to count 3.5 bn€ in over 600 deep tech investment deals in 2017.
As on many startup things, the UK is leading with the strongest AI community, Germany, France and Spain are following closely and actually catching up. Switzerland has the most AI startups per capita and most data analytics startups settled in and around Zurich. Most of the Top 100 research institutions for AI are in Europe, too.
Data Analytics companies make up the bulk of AI firms in Europe, perhaps due to AI needing data and its emergence from the Cloud and Big Data trends of recent years. Sales & Marketing AI firms tend to solve well-known problems with this new abundance of data, and take advantage of improvements in NLP (natural language processing) and NLG (natural language generation) within the mobile / IoT world. We therefore see many new companies in the fields of Text Analytics and Conversational Artificial Intelligence. Customer service bots and and personal assistants have been very popular verticals to create companies, supported by the advances of easily accessible APIs, for example.
Although robotics is one of the classic fields to use Artificial Intelligence, we are not yet seeing an corresponding increase in the number of robotics companies. Also verticals like logistics, education, and travel + Artificial Intelligence are getting more saturated.
Startups are the AI litmus test for regulators
(and regulators might not pass)
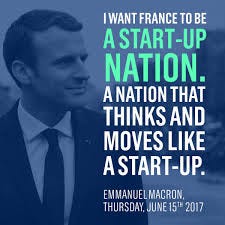
It is fair to assume that there would be less, much less, AI on the market without startups. No one but a startup has the balls to develop and bring tomorrow’s products on the market before corporates get interested. For policy makers this means that startups are the litmus test for all AI related policies, since they are the weakest link in the chain. If laws and regulations prove unfit for startups, the entire AI sector will suffer and eventually come to a halt.Restrictive policies will hit startups before having an effect on larger corporates and will become a bottleneck for innovation in AI in Europe altogether.
Where can startups get the necessary data to innovate?
First off, AI startups do not simply benefit from any data but from the right data, especially data that is cleaned and correctly categorised. So the trick is not the overall quantity of the data, but the quality of the data as well. They can compete against big players by building the right product on top of relatively small data sets that grow over time. The fact that investments in AI and M&A’s are on the rise is proof that there is demand for what startups are doing.
But how can a startup working with images, text or voice recognition gather the necessary data outside their user base? In order to get to the right training data, the ability to mine data online is key. Using common crawl, custom crawlers, bots or scrapers are great ways to make 9.5 labelled images on Google or 4.5 million labelled YouTube videos something like a common good. Data mining is the most democratic way to allow startups to climb the ladder without intruding on proprietary data.
Data mining is one of key drivers of the data economy. It allows startups to enter a market and generate value without harming other uses or exploitations.
It’s a great example of the non-exhaustive and non-harming use of data. Other rich data sources for startups are sensors and IoT devices that are either deployed directly, given access to by their users.
This is a huge opportunity for startups leapfrogging in niche areas. Examples for that is a vivid legal-tech scene in Germany or HR related services like source{d} or Seedlink.
How can startups test new AI without going getting lost in red tape? Skills and best practices needed.
A contender to becoming a top 10 question is: How can legislation accommodate testing and pivoting businesses? Airbnb, Uber and many others are proof that in the absence of legal room to innovate, moving fast and breaking things is the only way.
Regulators say: Get permission first.
Startups think: Ask for forgiveness rather than permission.
This may be reasonable for services and products that impact public safety, but seems excessive for other data applications. What if permission takes several months but a product or service iterates and improves after six weeks of testing? Regulators should allow for a synchronised or light-touch approach, such as in regulatory sandboxes or policy experiments, instead of front-loading.
What should I tell my elected representative about AI?
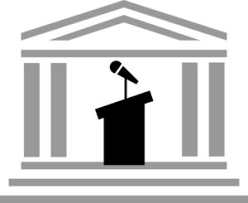
It is natural that groundbreaking trends like AI draw the attention of policymakers. The attention some AI applications attract will impact the way we look at liability, transparency, decision making and jobs. But regulators often get a tainted image of problems rather than positive stories. Stories of technology helping the blind to see or the 86 million Pineapple fund need more attention from regulators.
Bottom line is: Startups are the best examples of how Artificial Intelligence can do good and regulators should not take decisions without startups at the table. This is something Allied for Startups has tenaciously been advocating for. When policy tries to address fast moving technology, the first question must be whether there is an actual need for legislation. If its not broken, why fix it? And if there is a problem caused by technology, what is the best way to address it? These questions are unanswered when it comes to AI and yet policymakers are eyeing legislation. This allows a healthy dose of scepticism.
One the one hand Europe is home to some amazing universities and research hubs for AI, but on the other hand we still have a long way to go. Finding the right talent that has the digital and scientific expertise plus the ability to solve complex problems in our everyday lives, is key. Moving forward Europe will have to keep tabs on this talent by convincing the rockstars of today that it’s worth staying.
Without a doubt, startups are the most powerful vehicles of innovation. The most important AI innovations were once startups. To keep the pipeline open for new technologies in Europe we need to make sure we create the best possible environment for founders. We must allow startups to be born in Europe, and also to scale and gain traction right here. A big part of that is letting them experiment with technologies and explore the potential of AI without running into unintended hurdles.